Data-Driven AI transformation for Insurance
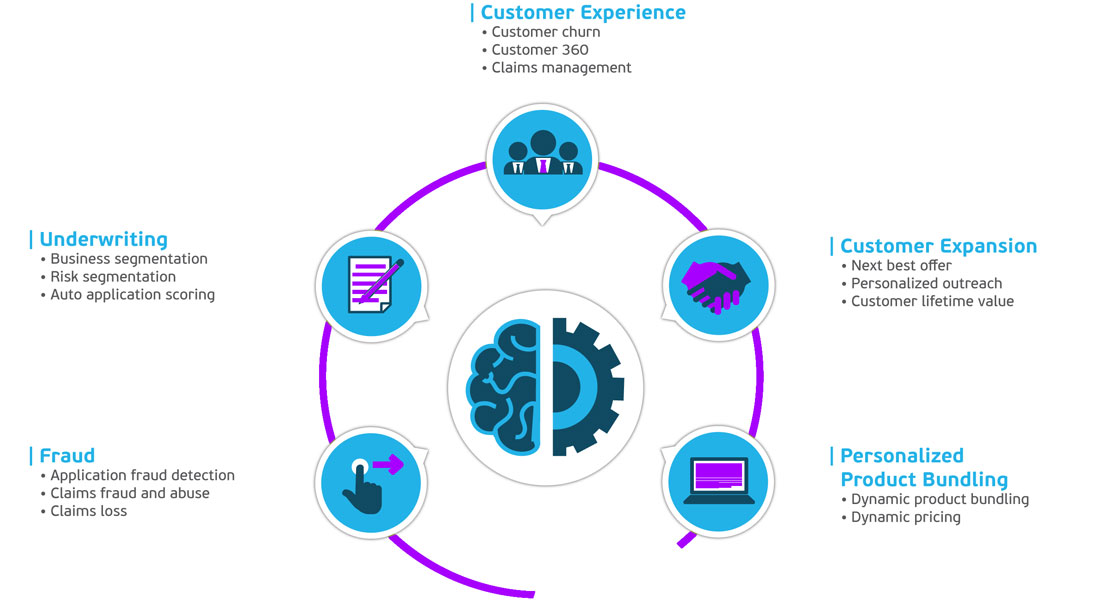
Data analytics has been constantly changing the way the insurance industry operates.
Today more and more insurance companies world-wide are willing to leverage the benefits of insurance analytics in their everyday business.
Covariance team enables insurance companies, in the present volatile environment, to find opportunities that deliver profitable growth while protecting and optimizing their enterprise by analyzing large, complex datasets with the use of advanced analytics and machine learning, and by integrating real-time analysis into existing systems and processes.
Detecting and building customer relationships and managing risks are key elements of growing profitable business and Covariance, by exploiting advanced analytics, machine learning and AI, can transform the way insurance companies operate. Covariance supports insurance companies to reap the benefits of increased customer satisfaction, increase loyalty, maximizing customers’ value, minimizing claims’ risks and costs, identifying, and minimizing fraud and improving underwriting and risk assessment processes.
Use cases
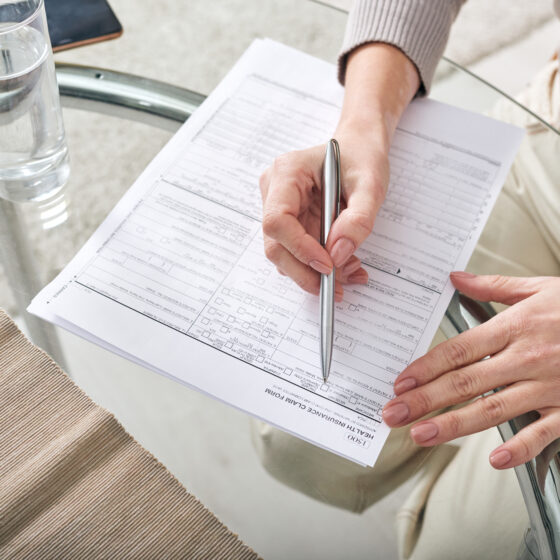
Claims Management (Streamlining the Claims Process with Data Analytics and AI)
Goal
Process Improvement → Faster Assessment & Fraud Detection → Customer Satisfaction → Increased Revenue and ROI
Challenges
Oftentimes, situations that arise from loss events awake the insuring populace towards the need for protective measure. However, claims are noted as the most critical channels and a defining link that shape the overall perception of the customers towards their insurer. According to Capgemini, the claims processing is the mirror to the customer that enable the insurers’ drive at improving customers’ acquisition, expectation, retention and business’ insight for product enhancement and company’s profitability. The assessment and payment of the claim should be fast and accurate to ensure customer satisfaction and prevent issues like fraud. Manual processing with analysts and rule-based systems making choices in claims can slow down the process results in a poor customer experience.
Opportunities
Data-Driven AI which is ideal for automating repetitive processes, can streamline processing by scoring claims for issues like fraud . Meanwhile, allows claims with low probability of an issue to be processed automatically while higher probability claims are routed to investigators for review. Data-Driven AI models can also supply justification messages for claim denials speeding up analyst decision making, and can be helpful to customers by informing them of issues with their claim, giving them the opportunity to fix the claim for re-approval and payment.
Application Specific Services
AI.R1 Solution.
Anonymization and Information Retrieval Solution.
From paper to Information solution.
Covariance Team supports you to “read paper”, create automatically structured data bases and acquire Information using Machine Learning Technology.
AI.R2 Solution.
Anonymization and Information Retrieval Solution.
Analytics, Insights and Reporting.
From paper to Insights solution.
Covariance Team supports you to “read paper”, create automatically structured data bases and harvest insights using Machine Learning Technology.
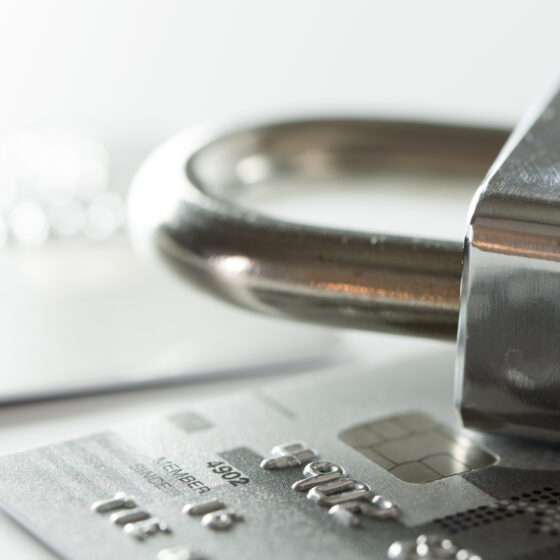
Fraud Detection (Detect and eradicate Fraud with Data Analytics and AI)
Goal
Fraud Detection → Strategic Advantage → Cost Reduction → Increased Revenue and ROI
Challenges
According to the FBI, the total cost of insurance fraud (non-health insurance) is estimated to be more than $40 billion per year, which costs average U.S. family between $400 and $700 per year in increased premiums. Insurance claims processing is dull, potentially leading to errors. Some fraud types are well known and can be located by using rules-based systems. However, new or more ‘’subtle’’ fraud is missed by rules-based systems until that fraud becomes evidence based.
Opportunities
AI ideally adapts to fraud detection for insurance claims. Machine learning models can be deployed to automate claims assessment and routing based on existing fraud patterns. This process flags potentially fraudulent claims in order to be further reviewed but also has the added benefit of automatically identifying good transactions and streamlining their approval and payment. More sophisticated detection systems can be deployed to find new patterns and to flag those for review, which leads to prompt investigation of new fraud types. AI systems can also provide concrete codes for investigators, so they can quickly see the key factors that led the AI to indicate fraud which streamlines their investigation. With AI based fraud detection, fraudulent claims can be assessed and flagged before they are paid, which reduces costs for insurance providers and helps reduce costs for consumers.
Application Specific Services
AI.R Solutions.
From paper to insights solution.
Covariance Team supports you to “read paper”, create automatically structured data bases and acquire knowledge using Machine Learning Technology.
Covariance Team supports you to identify fraud even from paper documents.
Application Specific Services
Signature identification.
Covariance Team provides you Neural Network based technology to automatically identify fraudulent signatures.
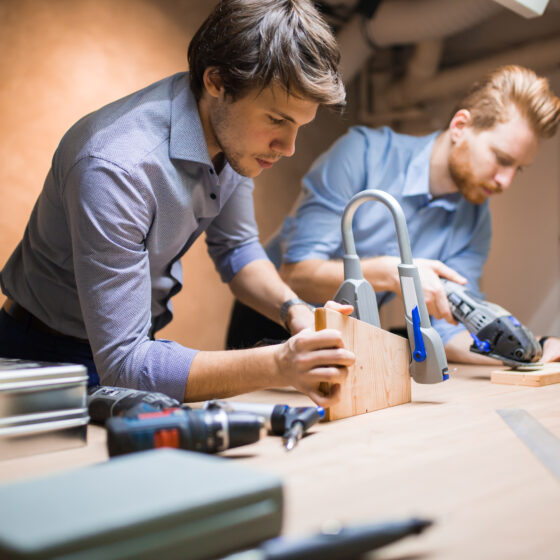
Personalized Product Bundling (Bundling the Right Product with Data Analytics and AI)
Goal
Strategic Advantage → Product Innovation → Cost Reduction → Customer Satisfaction → Increased Revenue and ROI
Challenges
Satisfaction improves among customers who bundle other policies with their homeowner’s policy, contributing to higher overall customer satisfaction with homeowner insurance companies, according to the J.D. Power and Associates. Specifically, 46% of customers who possess a bunch of insurance products, say they “definitely will” renew with their provider vs. 28% of non-bundle policyholders. With a variety of products to choose from, the pressure to retain customers, and consumer expectations for personalized treatment, marketing and selling generic products is no longer an option. For insurance companies this means that finding the right products to cross-sell into customers is a critical success factor.
Opportunities
According to an Accenture study, more than 80% of insurance customers are looking forward to a more personalized experience. Artificial Intelligence is ideal in recommending products and pricing in insurance. With Data-Driven AI models, insurance companies can do more personalized recommendations to consumer as well as to build the appropriate products for segments of clients by optimizing earnings and customer satisfaction. AI can also determine an individualized price based on consumer behavior and historical data. These recommendations can be used in web-based, call center and agent selling scenarios.
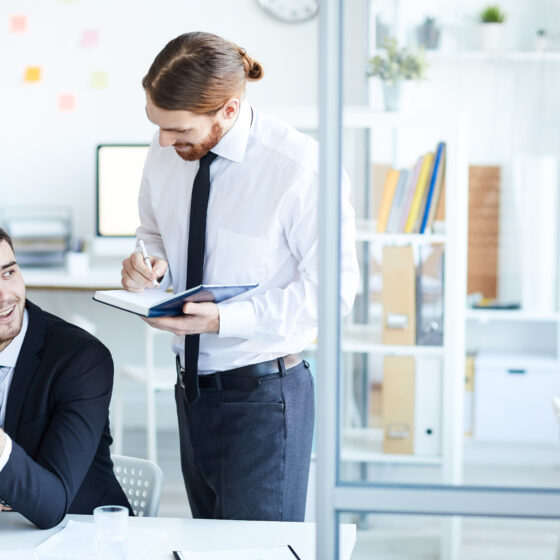
Personalized Rate Management (Customizing Rates based on Individual Data using Data Analytics and AI)
Goal
Strategic Advantage → Dynamic Pricing → Customer Satisfaction → Increased Revenue and ROI
Challenges
The vast majority of DNA tests link a certain disease to one or two specific genes. On the contrary complex diseases are known to be associated with a variety of genes, of which some are linked to other disease respectively. It is therefore sought to map all known associations and their correlation in order to achieve more accuracy in genomic analysis.
Opportunities
AI based analysis of disease-networks in a large-scale perspective
- Extraction of phenotypical knowledge from multiple sources using text mining/NLP.
-Identification and use of well-known biological databases.
-Creation of large disease-networks. Mapping and analysis of common features for drug repurposing.
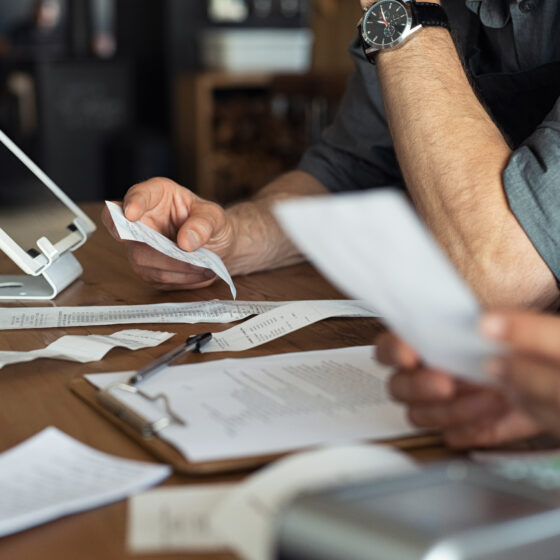
Underwriting Process Improvement (Customizing Rates based on Similar Groups using Data Analytics and AI)
Goal
Strategic Advantage → Cost Reduction → Increased Revenue and ROI
Challenges
The use of data is at the heart of each Insurance firm since decades,insurers have been using data in underwriting. Underwriting is the fragile method of assessing the peril posed by every client and coming up with a cost for the insurance policy, which is reasonable towards parties, insurer, and customer.
Opportunities
Data analytics and AI can help assess the risk category for every prospective client. This is also completed through clustering techniques taking into consideration not only individual scores for diverse risk factors but the correlation between these.
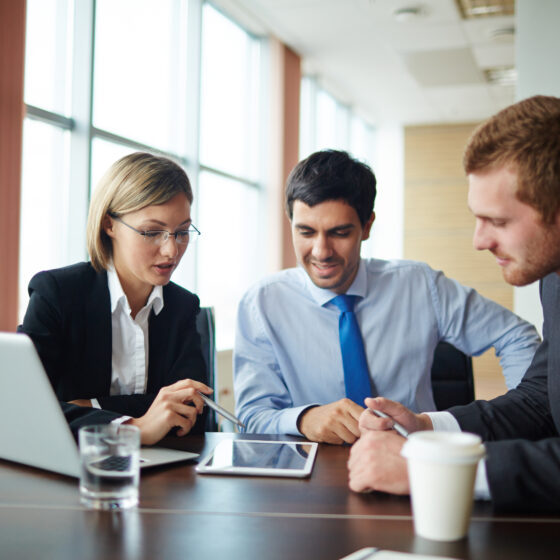
Customer Retention (Customizing Rates based on Individual Data using Data Analytics and AI)
Goal
Strategic Advantage → Customer Satisfaction → Increased Revenue and ROI
Challenges
The primary goal of any business is to stay in business. In other words, any business is aiming to retain customers for as long as possible, as it boosts the revenue of the company and increases the lifetime value of the customers. It is widely accepted that retaining a loyal customer in the Insurance industry is rather cost effective than acquiring a new one. Rule-based systems which is the traditional method for churn analysis, are inflexible and miss customers who leave, while at the same time generates false positives, ending-up giving expensive incentives to customers who do not need them.
Opportunities
Artificial intelligence and machine learning are the perfect solutions for customer retention and churn prevention. Data-Driven AI can deploy a variety of data, as well as new data sources and historical data, in order to determine risk. Artificial intelligence can also determine what type of promotional offer can achieve the best result for the highest engagement and retention for each individual customer. Based on this, the system can automatically personalize the promotional offer without the need of human intervention.
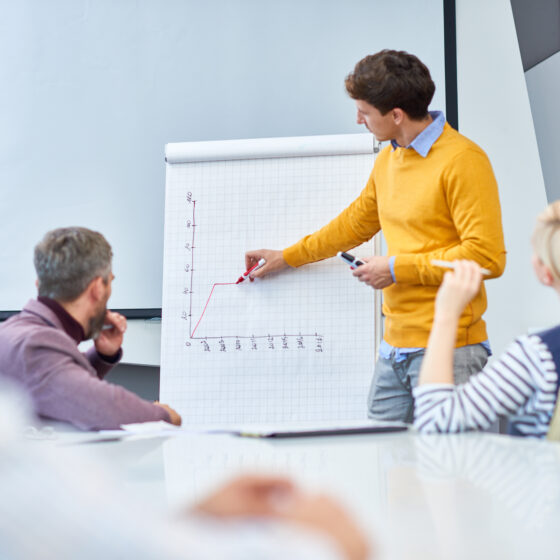
Enhancing Marketing Strategies (Advanced Customer Insights using Data Analytics and AI)
Goal
Strategic Advantage → Product Innovation → Cost Reduction → Customer Satisfaction → Increased Revenue and ROI
Challenges
Analysing customer patterns using predictive insurance data analytics boosts chances for insurers to cross-sales and up-sell policies. Services can be modified, product portfolios can become better by eliminating under-performing products and defining new ones, premiums can be amended, and targeted marketing strategies can be functional by insurers to develop business. Life, health, home, and vehicle insurance products can be customized to go well with the requirements of the customer. As a consequence it stimulates customer satisfaction and trustworthiness. Strong relationships with customers can be put up and churn charge can be eliminated. Customer achievement, which is usually the most significant product outlay for insurers, can be made even more efficient using smart and targeted marketing with a higher possibility of a switch to a retained client.
Opportunities
We use Data-Driven AI to target the right customers, identify dissatisfied customers, uncovering patterns of buying behaviors, and addresses customer service issues faster by correlating and analyzing a variety of data. The deep insights stemming from this process points out what is all the more important to your customer and can help you establish a dynamic, comprehensive view of individual customers and segments, understand what makes them high lifetime value customers, and ensure customer engagements are consistent, personalized and relevant across all touchpoints. Use your customer data to:
- Understand the way your customers behave and react and let this understanding help drive your decision making.
- Correlate the right product to the right customer.
- Listen to what your customers have to say and use this information to improve your services and become more efficient.
Customer Insight can be used to inform strategy and policy, to allocate resources, to manage performance, to market services, to change behaviors and to inform service design.
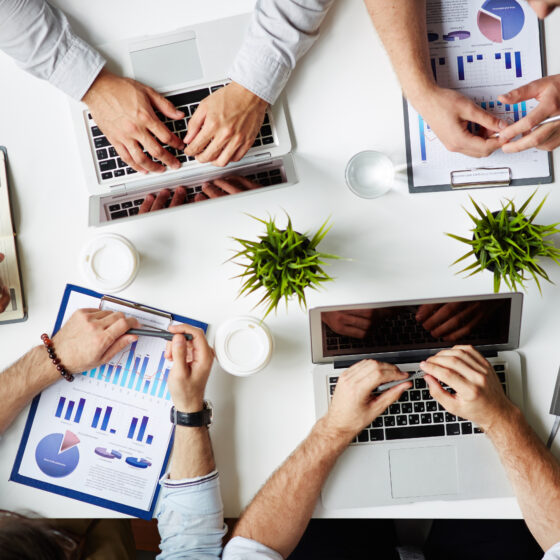
Sales and Channel Assessment (Data-Driven AI Assess Sales and Sales Channels)
Goal
Strategic Advantage → Cost Reduction → Customer Satisfaction → Increased Revenue and ROI
Challenges
It has been observed that customers tend to do more research using the internet, although ultimately rely on face to face interaction with the intermediaries for purchasing insurance products. Data-Driven AI can help insurers in deeper understanding of these distribution channels and help in devising suitable channel management strategies.
Opportunities
Data-Driven AI is a great solution for: Channel Strategy Optimization since it can help in analyzing the performance of different channels across multiple geographies, product lines and provide drill down facility to the level of a producer. An appropriate channel strategy in terms of business expectations, product mapping and payout structure can be framed and structured based on the Data-Driven AI.
- Sales Optimization since a comparison of business achieved as against the target, Geography wise, channel wise, product wise new business can help in identifying the trends across channels and can help in initiating mid-course corrective measures to achieve the planned profitability.
- Channel Analysis since insurers can compare the performance of various distribution channels and can drill down to the level of individual agents and products. The performance should be tracked closely and compared over a period to assess the effectiveness of developmental actions initiated.
- Channel Profitability since assessment of channel profitability can be done through a comprehensive analysis of new business, commission, persistency and claims by each of the channel and this can lead to redesigning the compensation structure, revisiting of products, revising of underwriting processes.
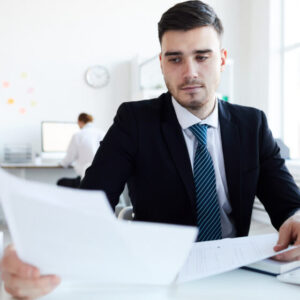
The Intelligent Broker (Make more informed business decisions with data-driven insights)
Goal
Strategic Advantage → Cost Reduction → Customer Satisfaction → Increased Revenue and ROI
Challenges
Winning companies are improving services and offerings through advanced analytics that leverage data inputs and outputs to predict future events (predictive analytics) and how actions change an outcome (prescriptive analytics). These insights help brokers spot opportunities in improving prospecting/cross-sell based on propensity to buy or introduce risk controls that will reduce future losses for their clients.
Many brokers provide access to both structured and unstructured data. Third party sources are providing a deeper level of information to brokers such as demographics, exposures, prior coverage, buying behavior and claims. The culmination of data storage and third-party data access enables brokers to better use analytics for enhanced business insights.
Opportunities
- Descriptive analytics provide insight into what happened in the past, such as in pricing and coverage benchmarking using “like them” cluster analysis.
- Automation solutions have become more intelligent. They can be applied in combination with optical character recognition (OCR) and more advanced natural language processing (NLP) solutions to extract data from forms or e-mails, execute manual data entry into systems or templates, and complete rule-based manual processes in core broking administration or billing systems.
- Secure optimal price by Leveraging external market data to determine market prices.
- Deepen relationships by using machine learning to estimate propensity to buy and suggest appropriate products to cross-sell.
- Gauge sentiment by incorporating the Internet of Things to identify potential churn and engage with clients to improve retention.
- Improve loss control by real-time monitoring of internal and third-party data sources to identify risks to recommend preventive actions to limit and/or eliminate losses
- Design optimal coverage and program structure: Using machine learning to suggest optimal coverage, limits and deductibles
- Enhance customer service: Automatic issuance of certificates using virtual assistance